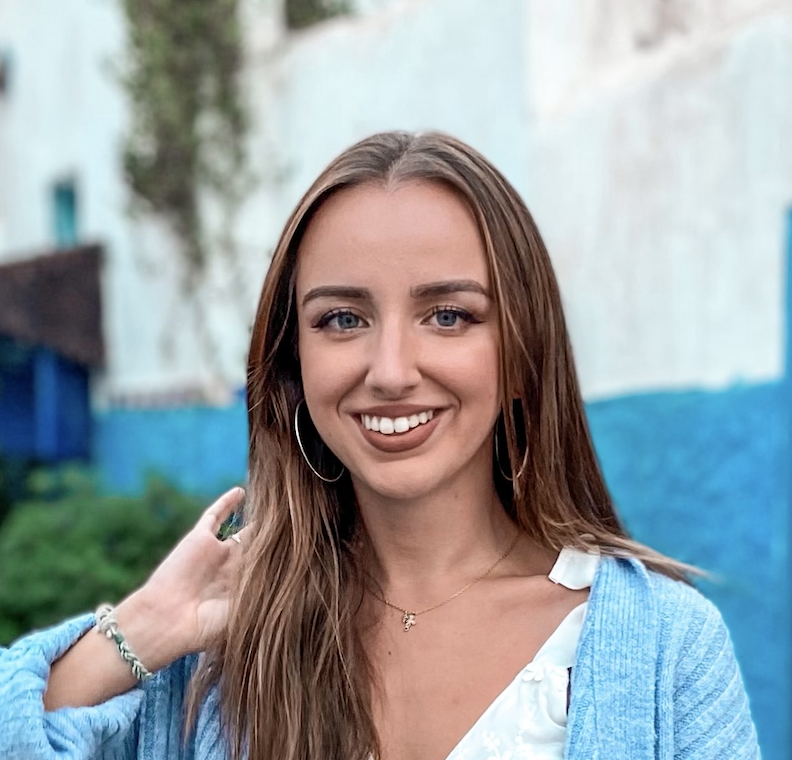
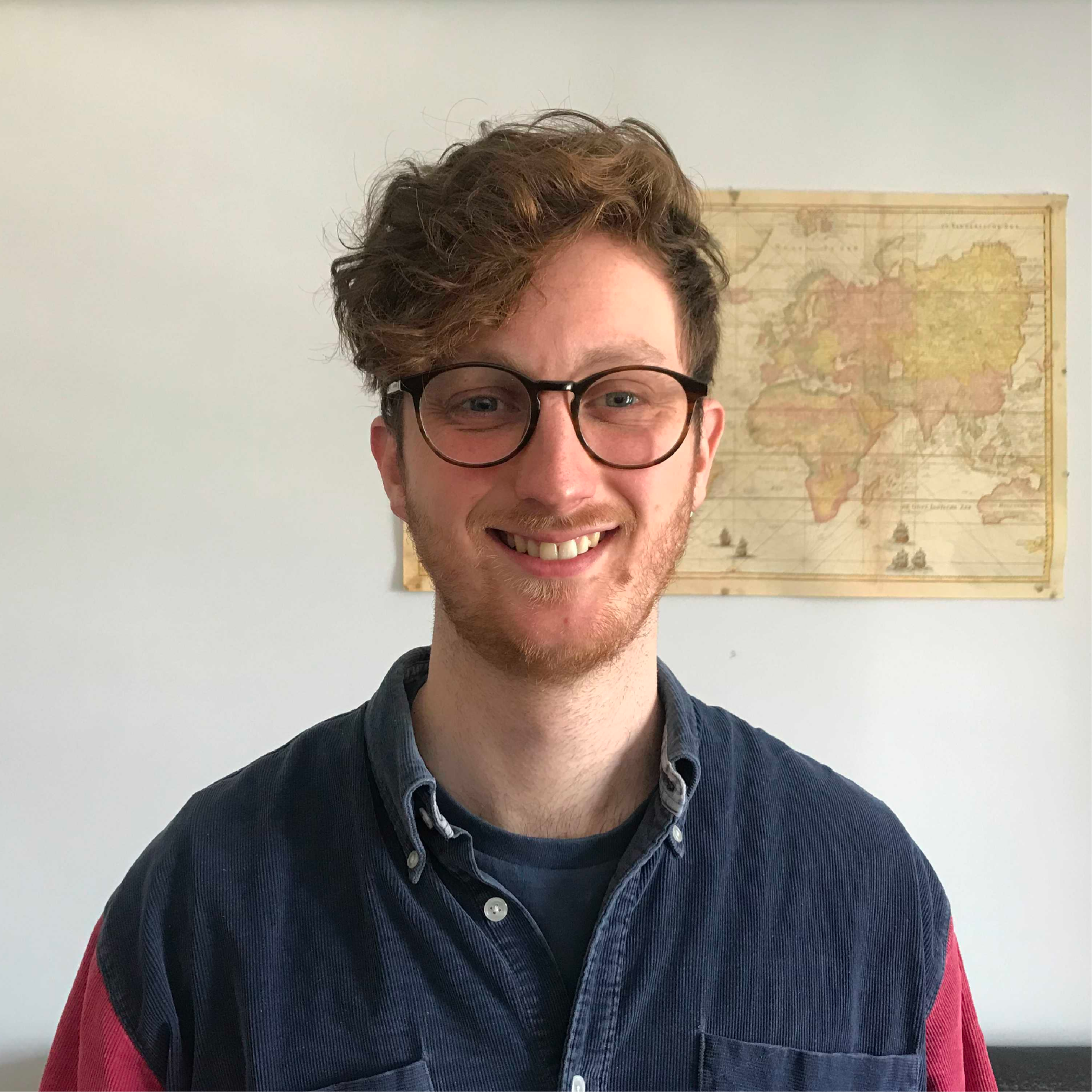
Finding the 'Perfect' Sales Candidate Using Clustering and Prediction: Graphext and "The Sales Acceleration Formula"
The Sales Acceleration Formula is written by an MIT engineer who found himself as employee number 3 at Hubspot, faced with the task of creating a sales operation from nothing. Growing sales from $0 to $100m in 7 years, the book documents Roberge's approach to building his team using the mindset of an engineer. The author writes about the different characteristics of successful salespeople, trying to uncover them in hiring processes as well as developing the top-performing sales formula for specific businesses. Naturally, our sales team found themselves considering how to improve this process using Graphext.
Our team created a fictional dataset of 100 employees, each with a rating for a set of key characteristics.
Without fear of endangering their own jobs, our team started to apply a data-driven approach to finding the ideal candidate for sales roles. With the belief that it would be possible to find relationships between successful salespeople and their attributes, they started by creating a fictional dataset with records for 100 employees, each with ratings for a set of 10 characteristics. With the fate of each imaginary employee's bank balance in their hands, they created a variable, 'work assessment', which featured a sales performance success rating for each employee.
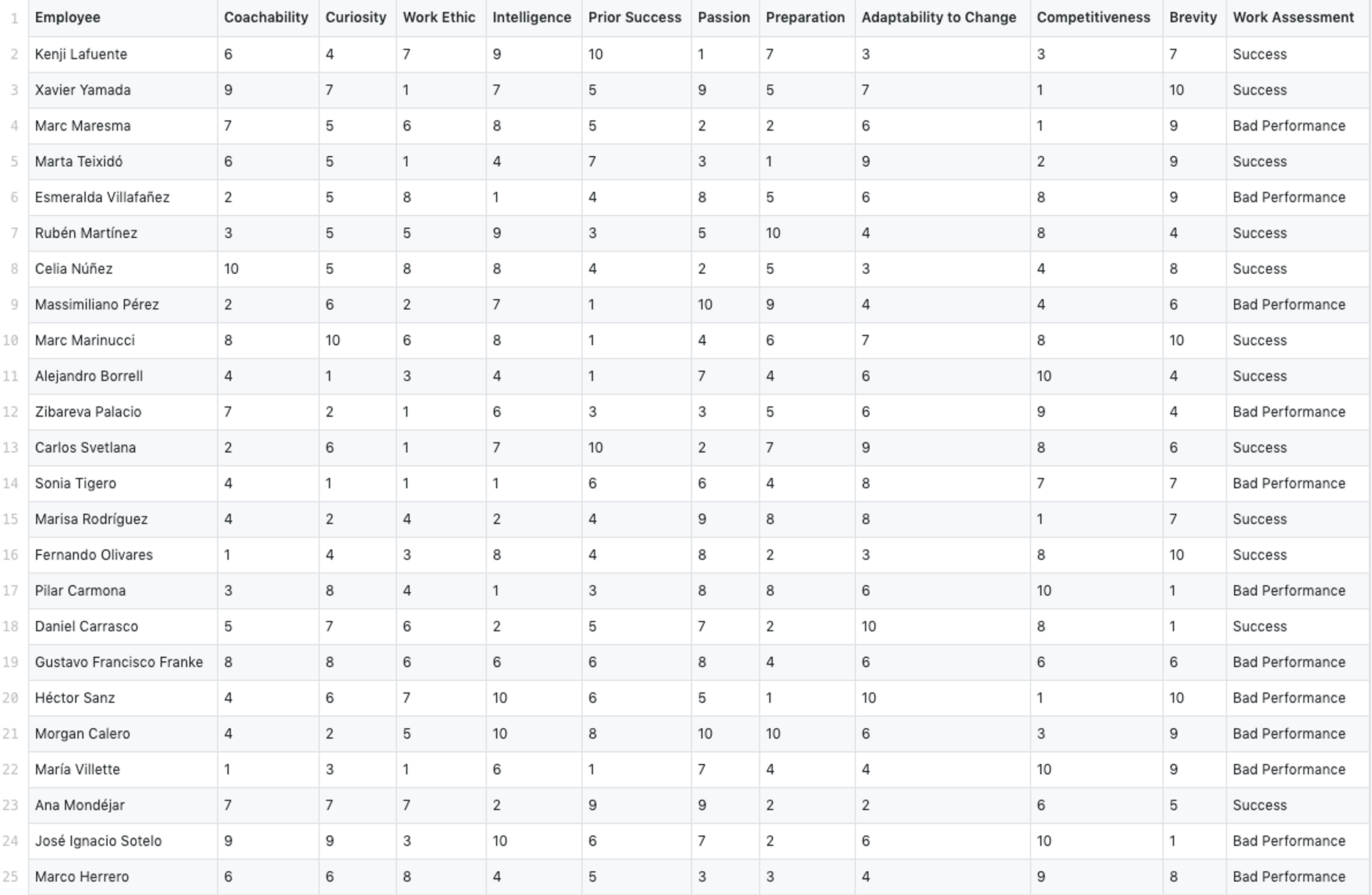
Fictional Dataset with 100 Employees, each given a rating between 1 - 10 for a set of 10 characteristics.
A Theory of Ideal Characteristics
The first task was to create a list of the personal attributes that our team felt were most closely connected with a successful sales record. Next, they needed to score each employee from 0 to 10 for each attribute. Whilst successful sales characteristics vary between companies and sectors, our team focused on a broad formula and decided upon the following 10.
- Coachability
- Adaptability to change
- Curiosity
- Passion
- Prior success
- Intelligence
- Preparation
- Work ethic
- Brevity
- Competitiveness
Demonstration of each of these attributes is an important part of getting a job as well as performing well in it. Nonetheless, not even top-performing salespeople can excel in each and every one of these departments. Our team wanted to demonstrate Graphext's ability to identify which of them are most strongly correlated with success, which don't seem to matter and whether there were other important traits that were not being computed.
Our team wanted to demonstrate Graphext's ability to identify the sales characteristics that could be strongly correlated with success.
To build the project, the sales team focused on clustering, a type of analysis linking data points based on their similarity, so that they would be able to find patterns connecting the fictional employees to one another. They added all 10 characteristics as factors in the analysis and listed work assessment, their success metric, as the target variable they wanted to gain a deeper understanding of.
Variables Correlated with Success
Since the characteristics in their dataset were all positive, the hypothesis our team worked with was that there would be certain attributes that were more relevant when considering successful sales performances. Additionally, none of our imaginary employees was given a drastically low score for any of the variables considering that if they were - they wouldn't have got the job.
The results suggested that coachability, competitiveness, prior success, adaptability to change and curiosity were the variables most strongly correlated with a successful sales record in the demo dataset. On the other hand, our team found that neither work ethic nor preparation were important factors, nor were intelligence or passion.
Although our team weren't working with "real-world" data, they were able to recognise a stronger correlation between certain variables and successful performance.
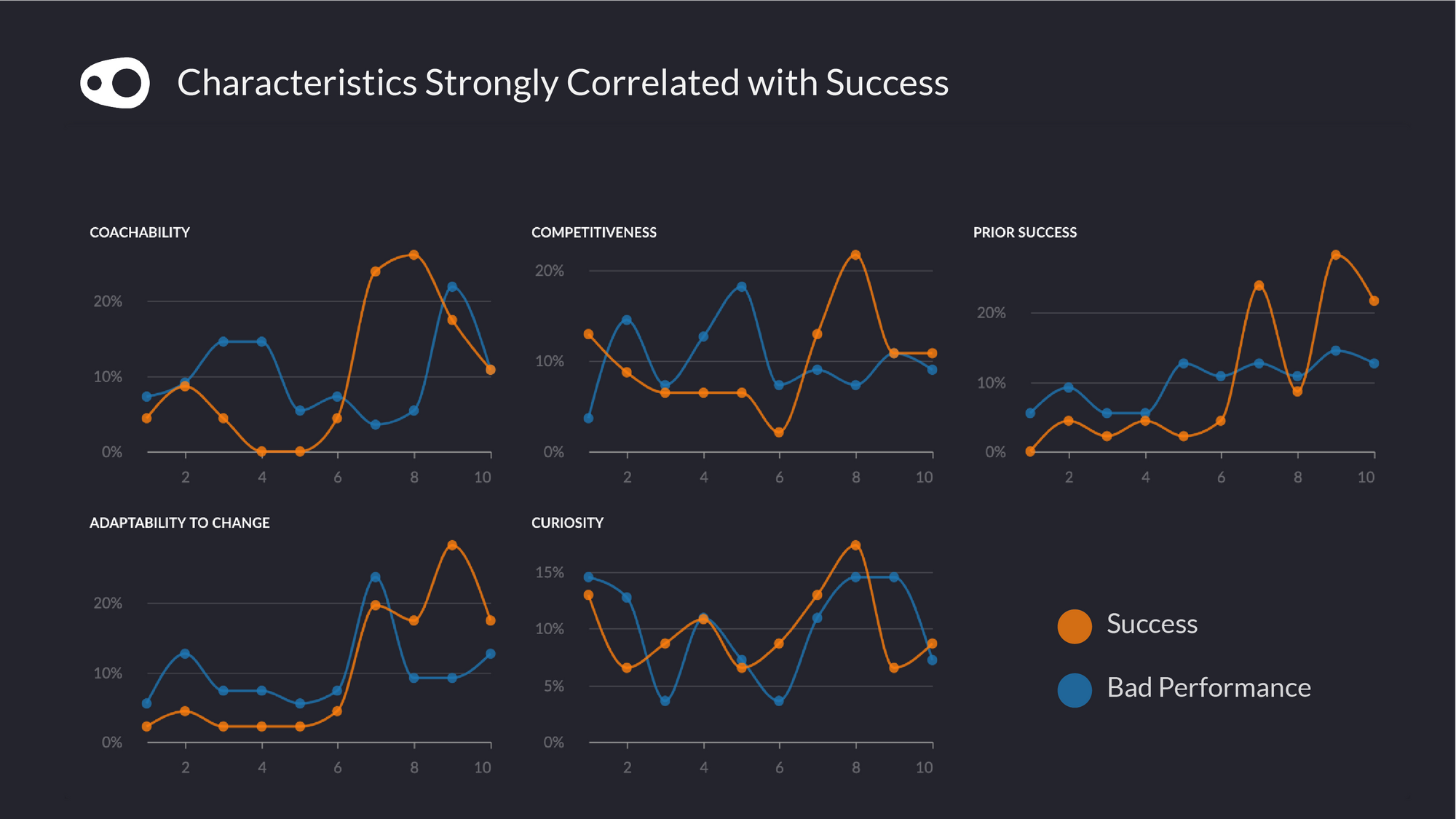
Compare: Characteristics that Graphext suggested were most strongly correlated with a successful sales performance.
Coachability, competitiveness, prior success, adaptability to change and curiosity were the variables most strongly correlated with a successful sales record in our demo dataset.
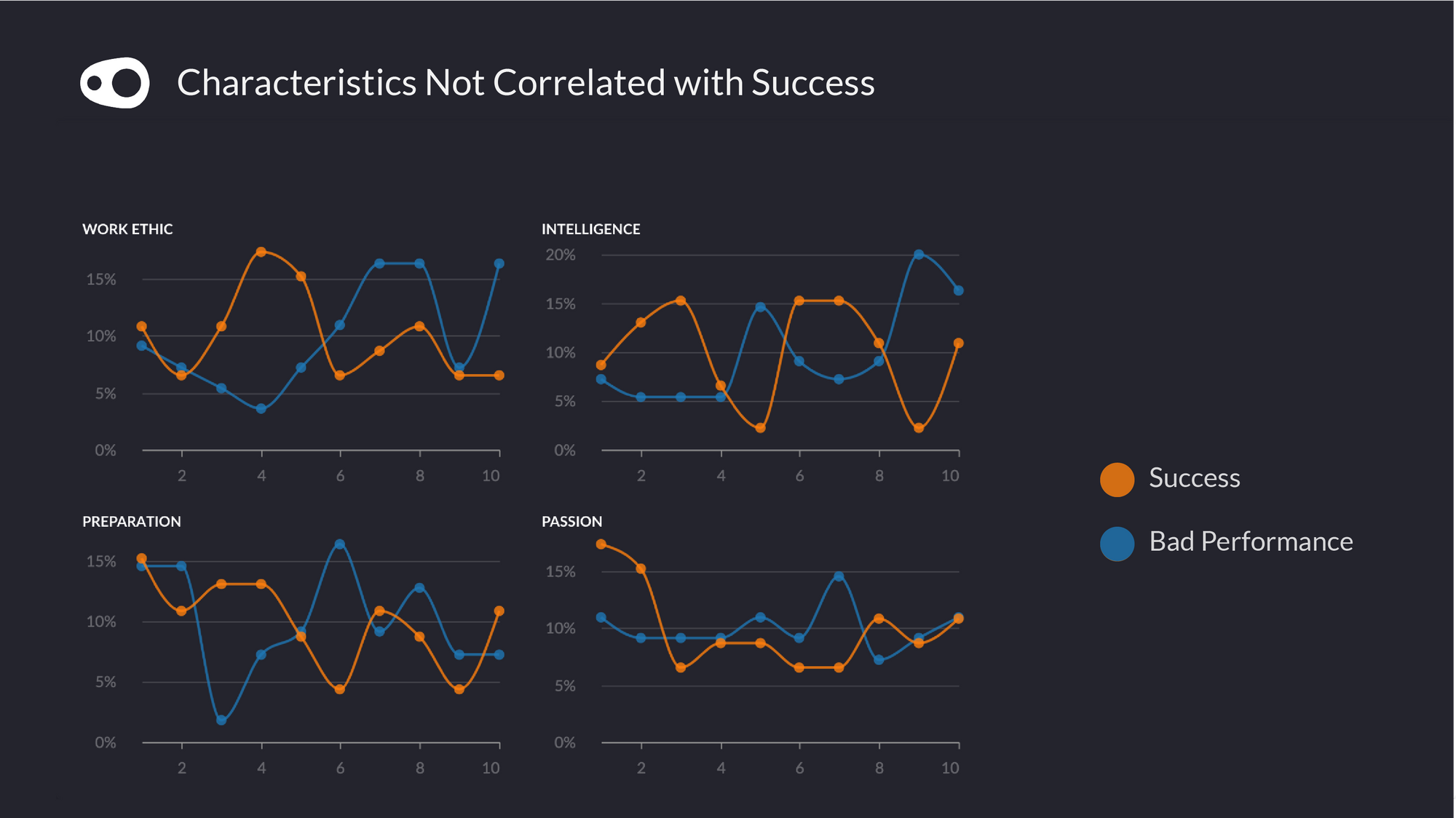
Compare: Characteristics that Graphext suggested were not correlated with a successful performance.
Key Clusters
In the cluster featuring the lowest number of high performers, we found that employees are extremely intelligent and present high preparation, coachability and adaptability. Nonetheless, none of these fictional employees possessed a high rating for our prior success variable, which our cluster suggests has significantly contributed to their poor performances. It's possible that these data points could have represented entry-level employees, presenting lots of qualifications but barely any experience.
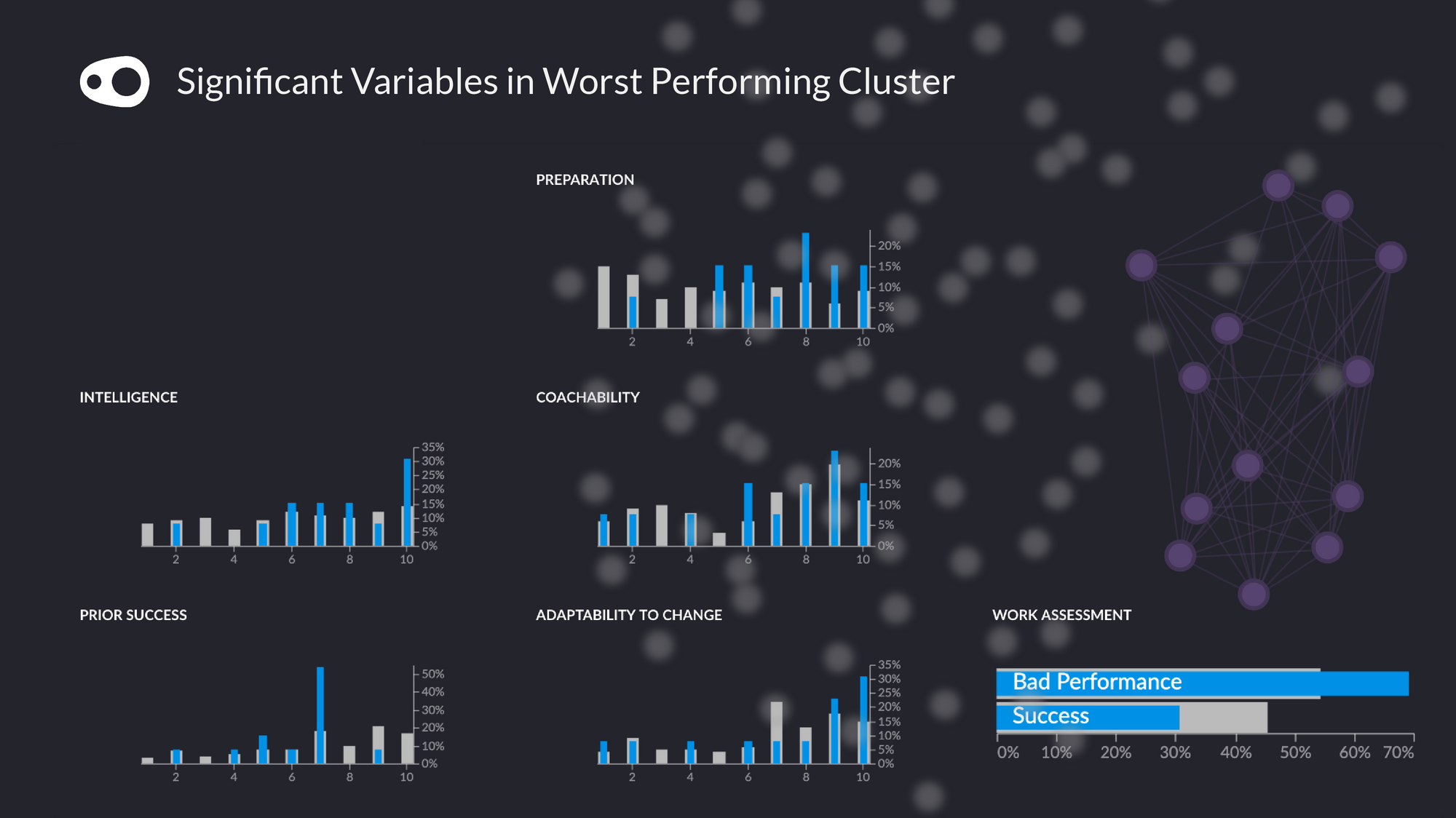
Graph: The cluster containing the worst performing employees.
Turning their attention to the cluster containing the highest number of high performers, our team were quick to identify that coachability, adaptability to change, competitiveness, prior success and work ethic were the traits most commonly shared by these fictional employees. Identifying that many of these salespeople had a low intelligence score was a discovery leading our team to hypothesize that intelligence is not as important as a history of prior success when considering top sales performance in the demo dataset.
In order to be a top performer within the realms of this fictional dataset, employees needed to be highly competitive.
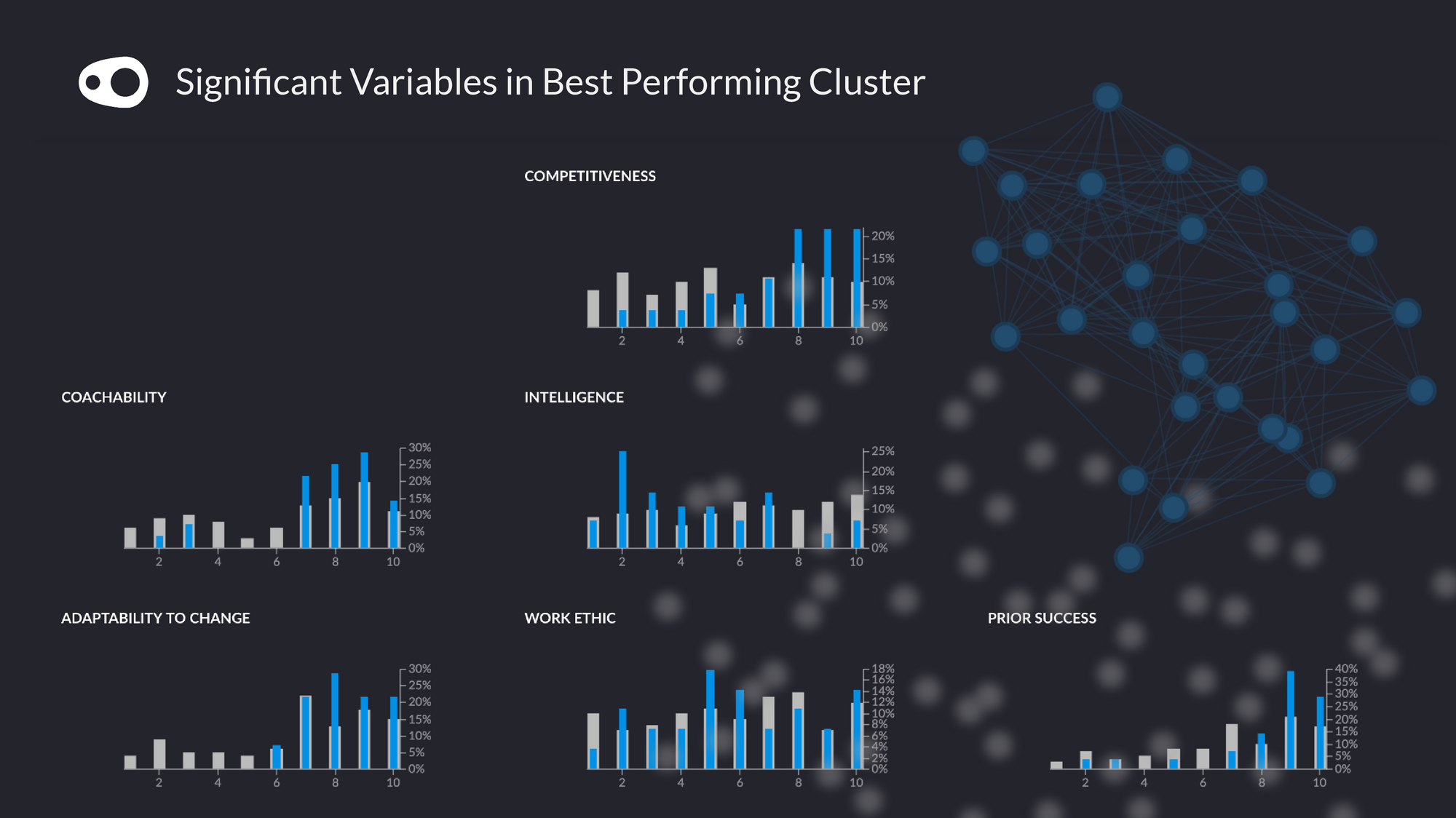
Graph: The cluster containing the best performing employees.
Predicting Sales Performance
Having identified the characteristics most strongly related to both successful and poor sales performance, our team began to consider whether it might be possible to create a model predicting the sales performance of our fictional employees based on their characteristics. In future, this model could be used to predict the performance of new candidates.
Using the same fictional dataset our team created a new project using a prediction flow, repeating the same process of adding the 'work assessment' variable as a target and the characteristic variables as factors. Graphext then created a model that predicted the 'work assessment' variable for each employee based on their characteristic scores.
Graphext created a model that predicted the sales performance of each fictional employee based on their characteristics.
Despite the dataset being limited to 100 employees, the model was able to make strong predictions and correctly identified the 'work assessment' variable for every fictional employee in the dataset. This identification is immediately reflected in the project's Graph, which groups the employees into two distinct groups; successful performers and poor performers.
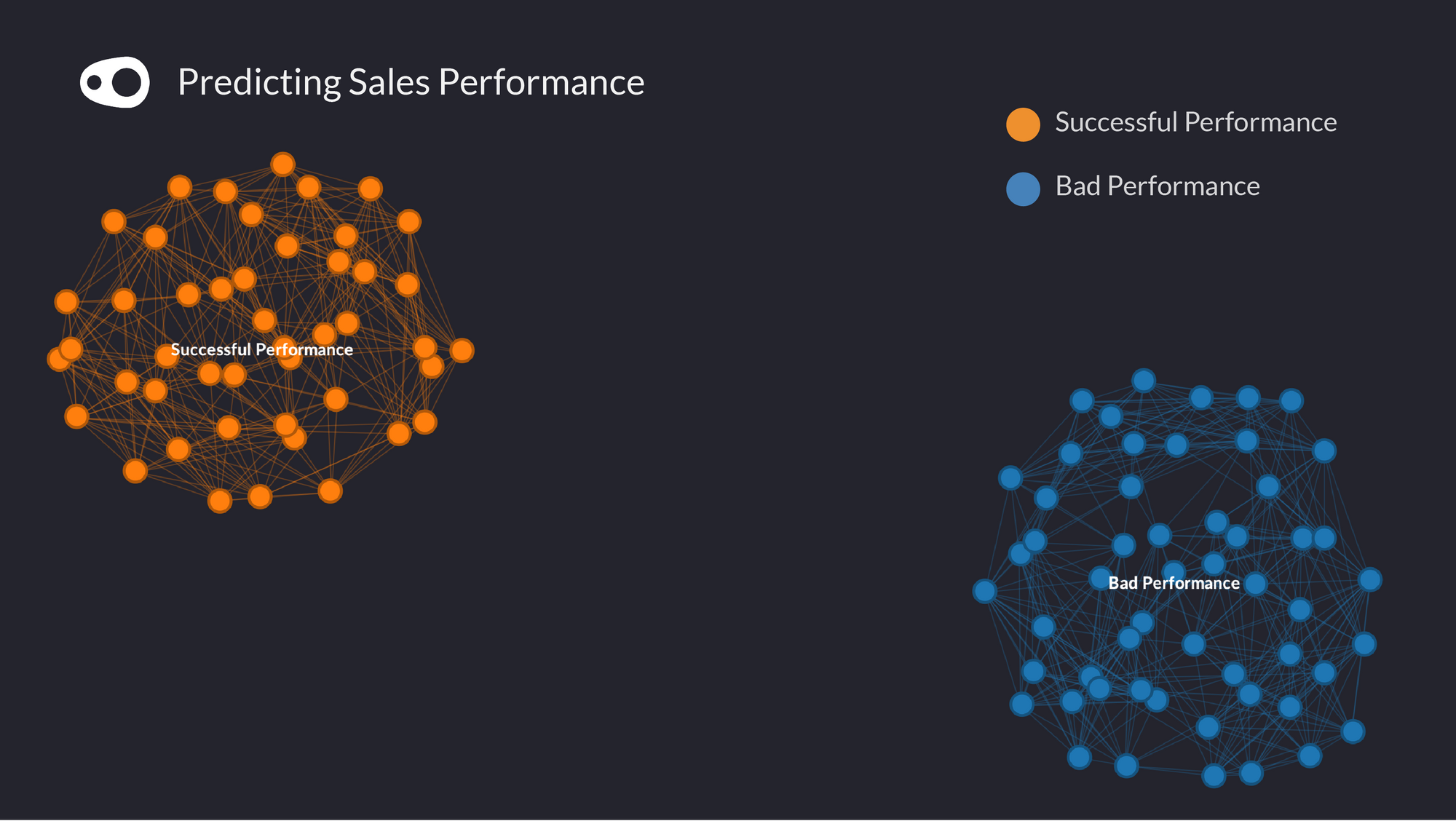
Graph: Our prediction model recognised two distinct groups; the successful performers group and the bad performers group.
On top of this, the prediction model recognised distinct clusters within each of the two groups. Differences in brevity, preparation and competitiveness scores appeared to be significant in distinguishing between the two clusters of successful employees. Recognising different types of successful employees is an important part of understanding that there is more than one way to succeed. Using a prediction model similar to the one our team created could not only help employers recognise whether a candidate has what it takes to be successful but also to identify the way in which they would become successful.
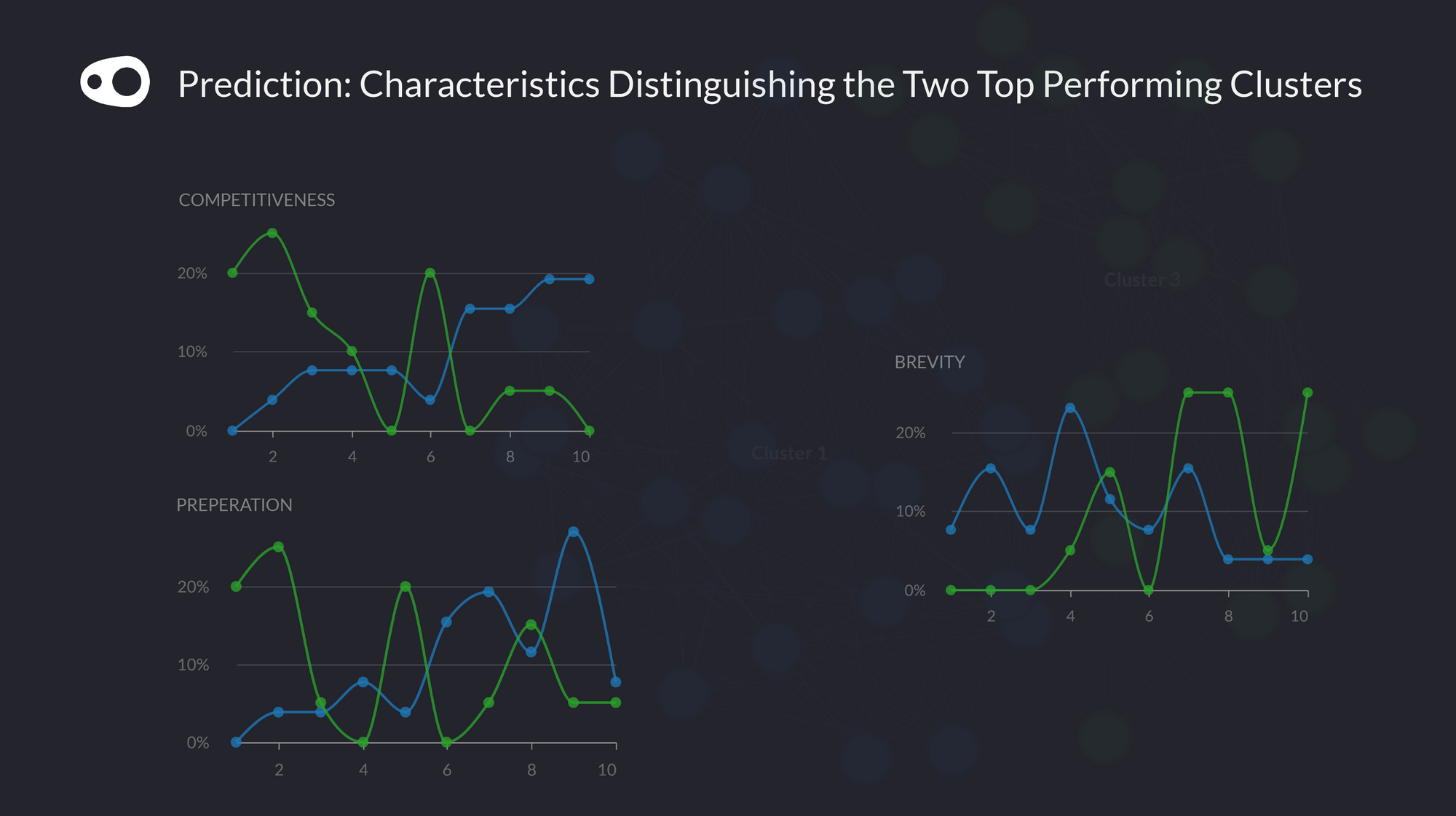
Compare: The characteristics distinguishing one cluster of top performers from the other.
Different Ways to Succeed
As Mark Roberge mentions in part 2 of his book, 'The Sales Training Formula', the best salespeople are individually great for diverse reasons. Salespeople succeed in their own unique way. Although new employees need training and exposure to sales best practice within their own companies, it is important to emphasize the need to allow them to be flexible in their journey towards success. They need to be able to use their own superpowers.
Sales superpowers are unique to each person. Each salesperson has their own journey towards success.
To demonstrate this, our team compared two fictional employees from the dataset. They visualised the traits of Ana and Alejandro and found that the two individuals were very different in many regards. While Ana is very curious, she's doesn't have a strong work ethic. In contrast, Alejandro is not curious but has a strong work ethic. These two salespeople reflect very different characteristics but are both top performers.
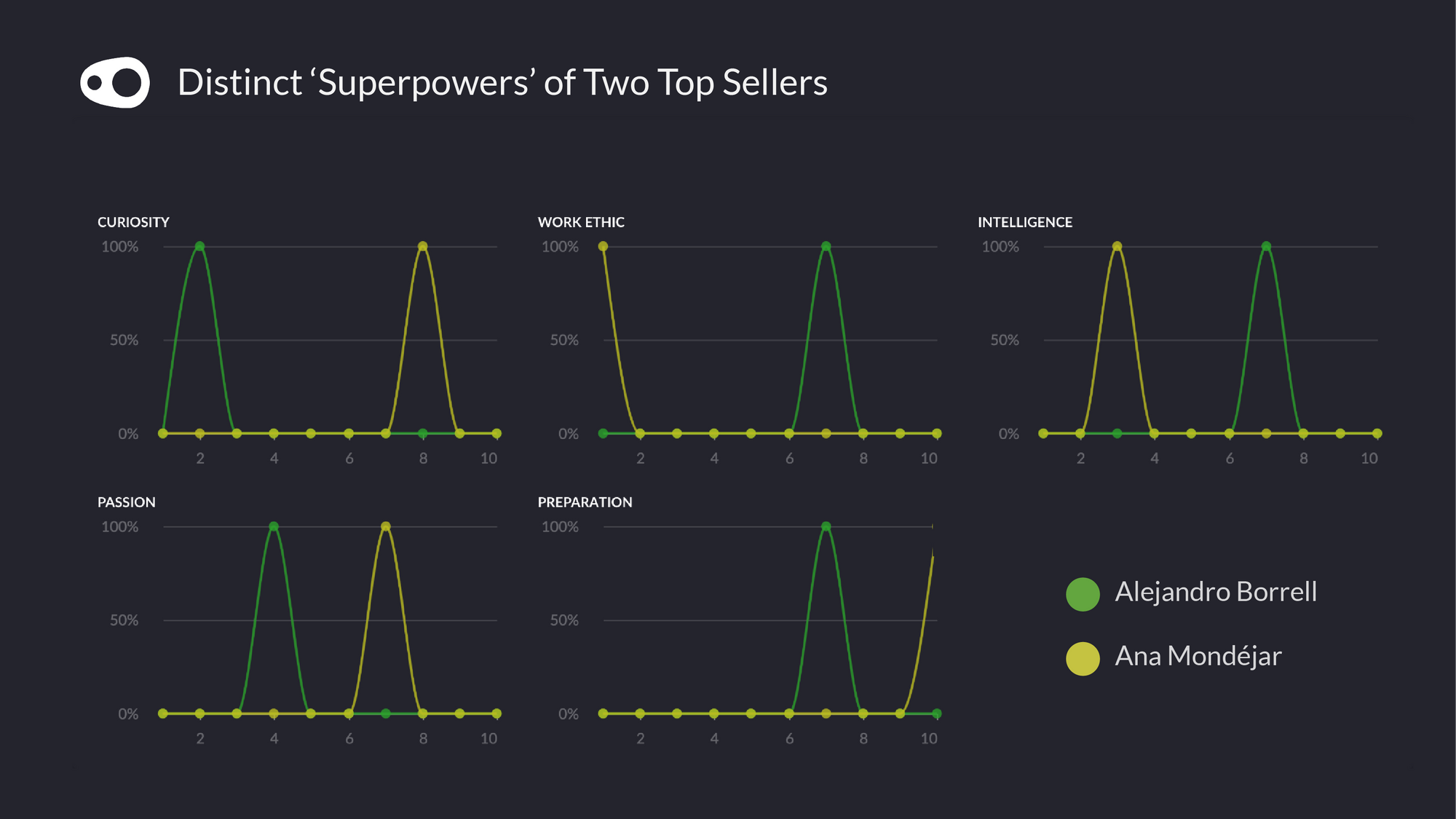
Compare: Two fictional employees with very different ways of succeeding.
As well as helping to identify which characteristics can be correlated with a strong sales performance, this kind of analysis might also be applied to employees from a range of different sectors. The workflow demonstrated by our team could not only be used to track the performance of existing employees but also to recognize the attributes that would be most important to look for when hiring new candidates.