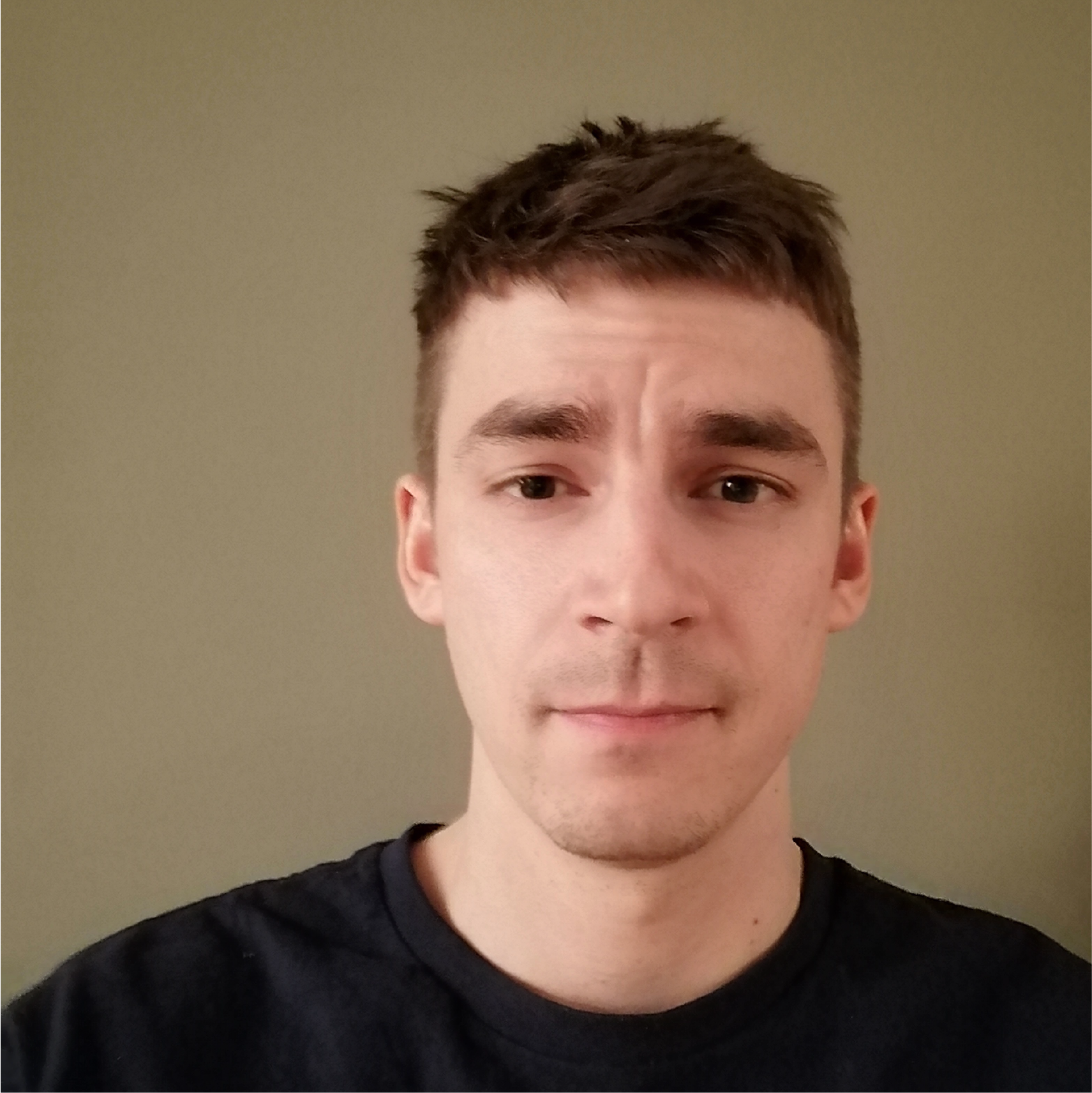
Good Risk vs Bad Risk: Deconstructing the Features of 1000 German Loans
In every business, it is important to understand your customers but no more so than in the world of finance - where the stakes are comparatively much higher. For a bank, each customer presents a risk especially when it comes to loaning money. Bad loans are full of risk, good loans are not. Ultimately, deciding whether that risk is worth taking is a decision of paramount importance and can affect the bank balances of people the world over.
Investment in risky loans is more likely to end up with customers defaulting on their payments - the consequences of this have been made painfully clear in recent history.
With this kind of responsibility at stake, banks tend to analyse their customer's finances with the utmost scrutiny. Loans are granted - only - when an applicant meets certain criteria. But for people outside of the financial sector, these characteristics often seem mysterious, tangled and daunting.
We were interested in finding the characteristics of loans that were most important to banks and lenders.
Aside from a person's credit history, there is a multitude of factors that help banks make decisions on the suitability of a loan. Factors including whether or not someone is a homeowner, a person's age, their direct debit history, and how much they earn all contribute to a risk profile.
But disclosure of these characteristics, how applicants are scored and which features make it more or less likely for loan applications to be approved, are not as transparent as many of us would like them to be.
Due to the privacy with which datasets of loan applications are protected, the challenge of finding a dataset to explore proved to be more difficult than usual. We had to change our angle ... and our time period. Putting on our beaded necklaces and frayed jeans, the team began to look into a dataset of loan applications ... from 1970.
Putting on our beaded necklaces and frayed jeans, the team began to look into a dataset of loan applications ... from 1970.
Our team decided to build a project using the features of an application to predict whether an applicant would have a good or bad risk rating. This meant reverse-engineering the features of a loan application in order to understand how they related to the loan's risk rating. With Graphext, you can do this using the visual editor in under 10 minutes.
Our model used Risk as its target variable and every other characteristic of a loan application as factors, clustering loan applications based on the similarity of these factors. Our intention was to uncover the constitution of high-risk or low-risk applicants.
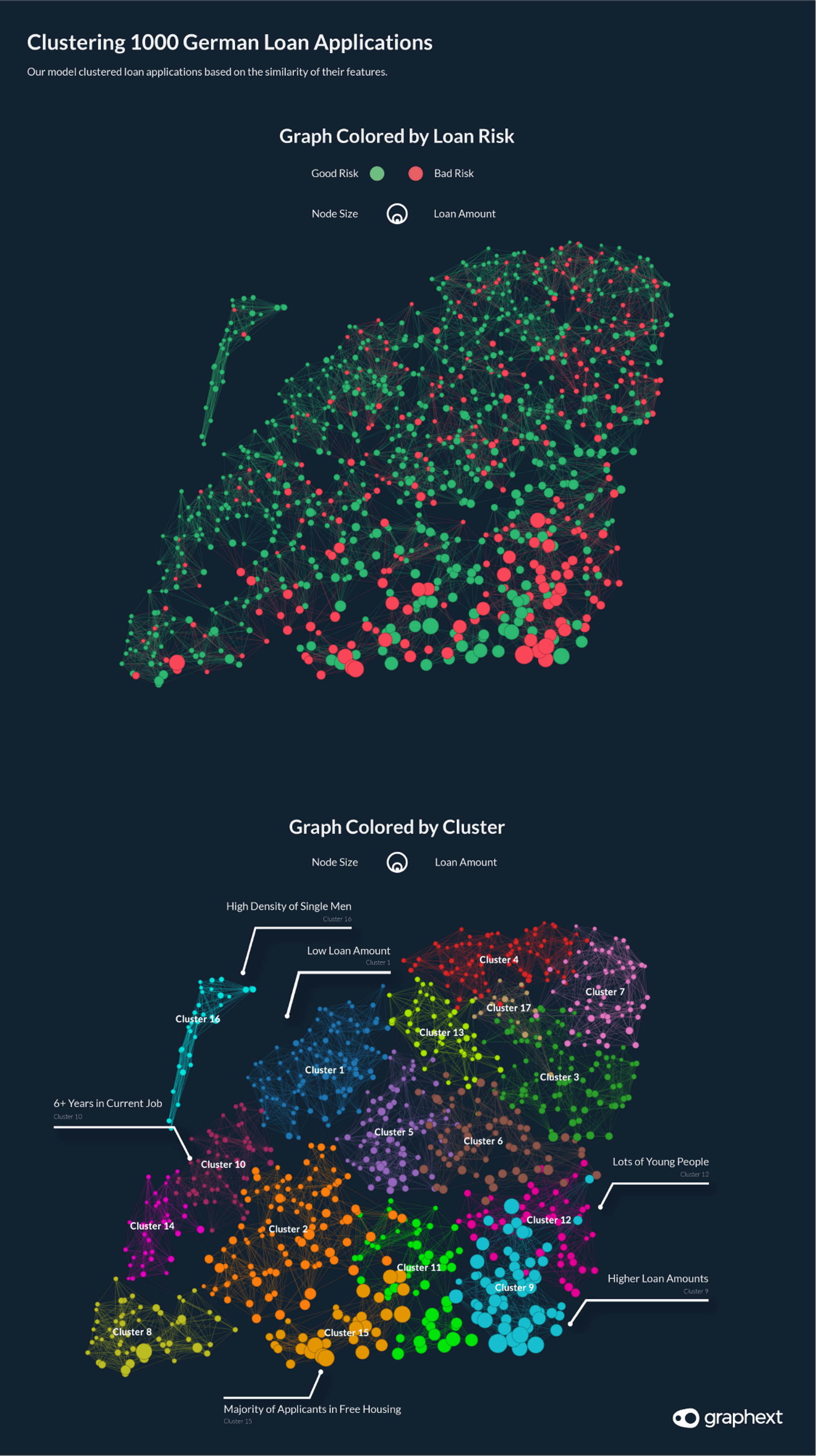
Graph: Clusters and Risk Value of 1000 German Loan Applications
A Successful Model
A model's error tells us whether the model was able to recognise a relationship between the factors and the target variables.
After executing the model, the team started to inspect the Graph, the network visualisation wherein loan applications were grouped together into clusters. A models accuracy - or error score - is important. This helps us recognise whether the model was able to recognise a relationship between the factors and the target variables.
The model we built had a low error score - 57 incorrect risk predictions out of a dataset of 1000 loan applications. It seemed to be clear that the model was able to understand which factors were most significant in leading to a risk rating. Next, using the Graph, we set about analysing the data to identify the factors most strongly contributing towards a bad risk rating.
Value Distribution in the Dataset
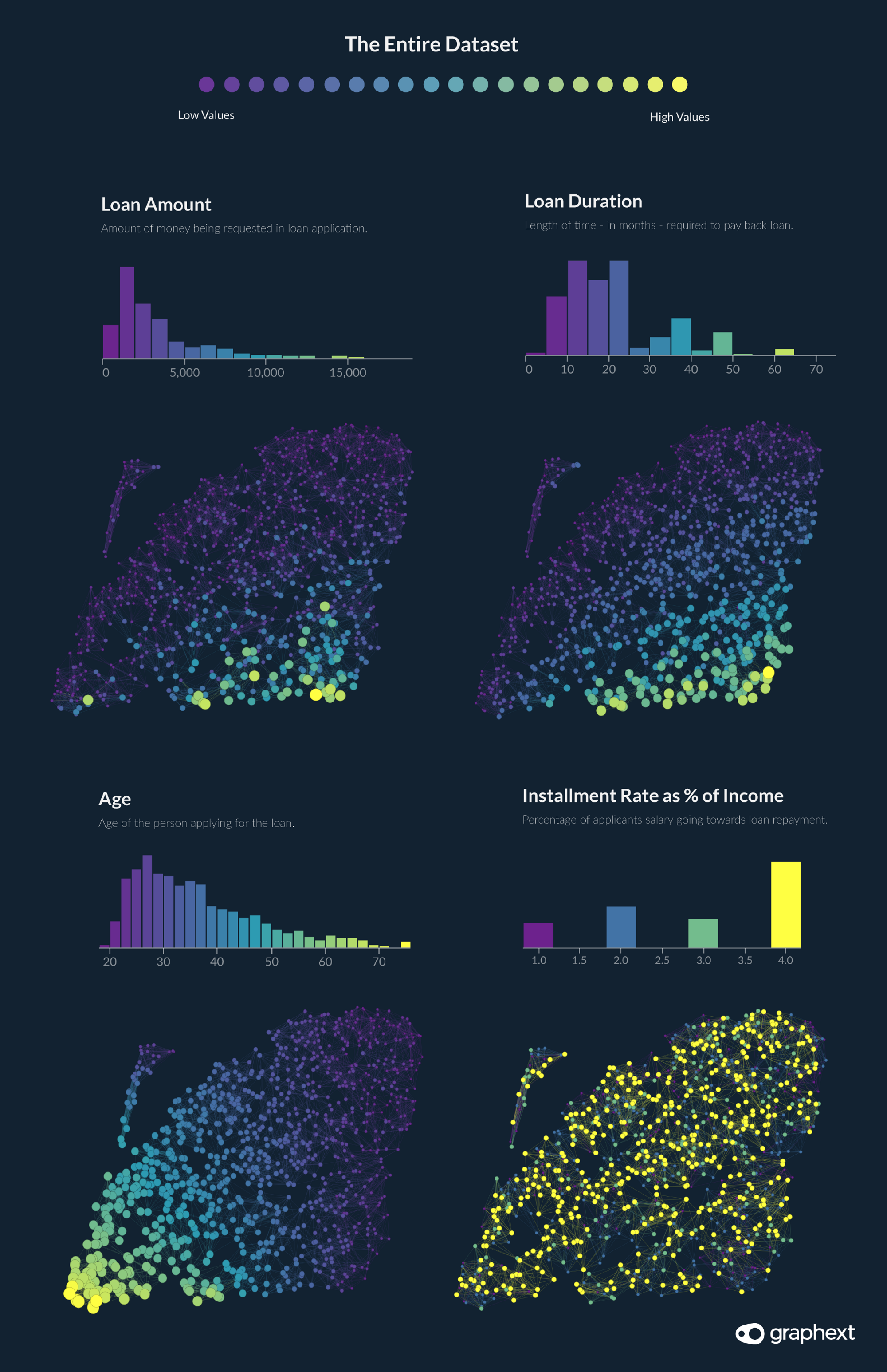
Graph: Distribution of quantitative values in the entire dataset.
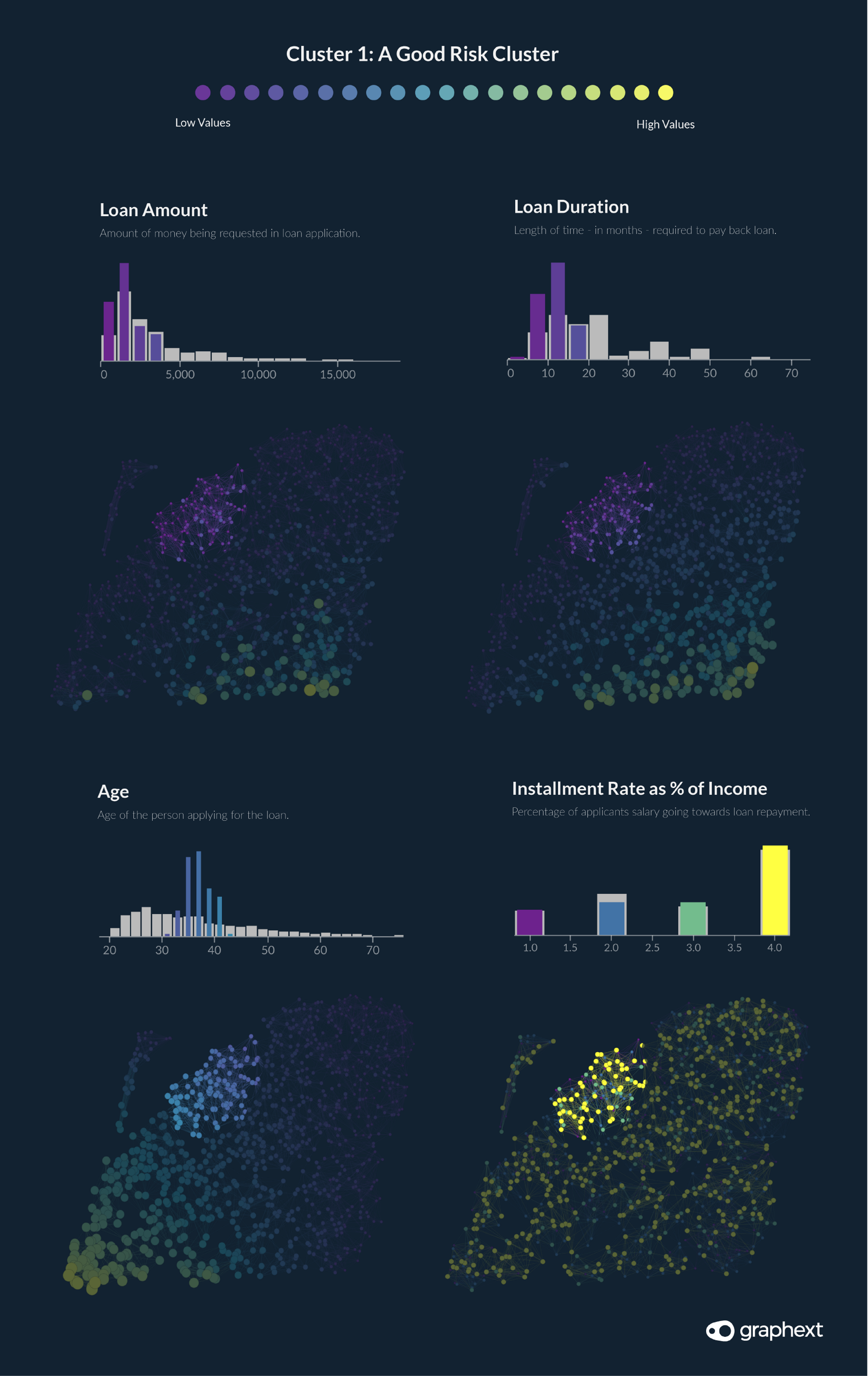
Graph: Distribution of quantitative values in cluster 1
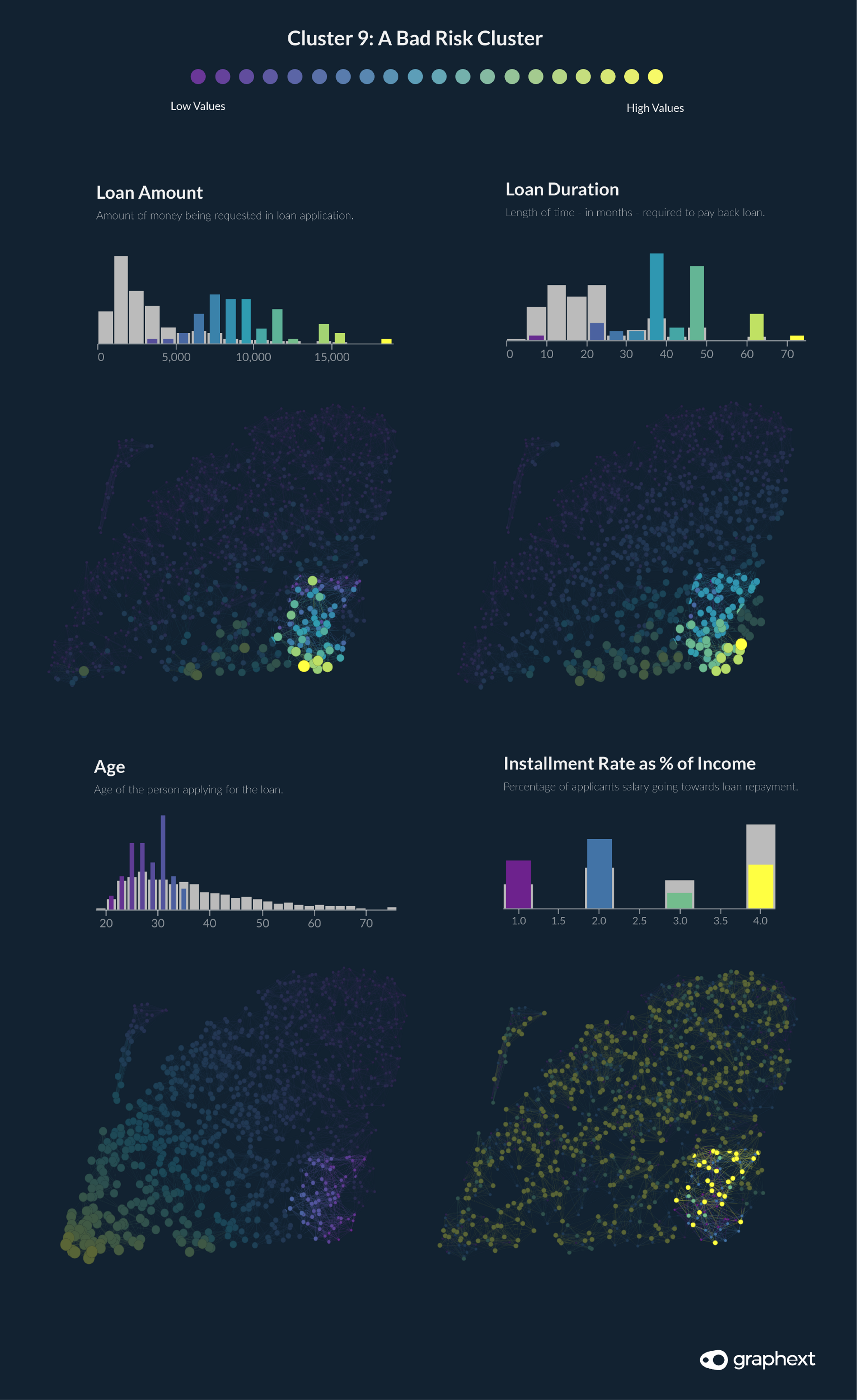
Graph: Distribution of quantitative values in cluster 9.
Cluster 9: A Bad Risk Cluster
With Cluster 9 making up just 5.6% of the dataset but having double the average score for bad risk, we started to inspect this important cluster in order to analyse the makeup of a bad risk applicant.
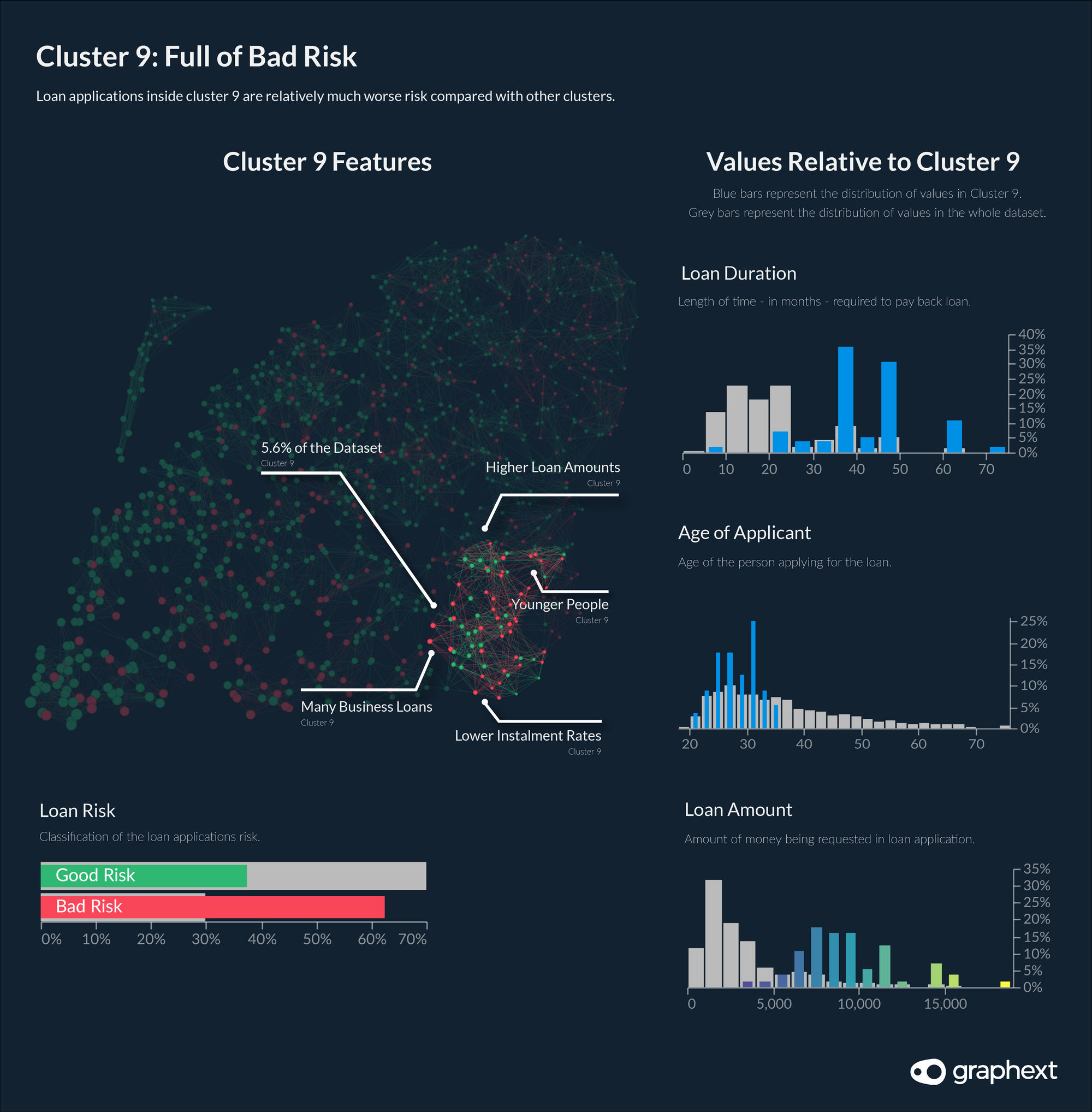
Cluster 9: The features of a bad risk cluster
After filtering our project to select data inside cluster 9, we could explore the features of this cluster dynamically. Cluster 9 loan applications are for higher sums of money and for long payback periods with smaller instalment rates. Moreover, applicants are generally younger people between the ages of 20 and 36 looking to start a business.
Cluster 1: A Good Risk Cluster
In order to evaluate the reverse of this, we turned our attention to Cluster 1 - a cluster containing the highest number of good risk applications. It made up 10% of the dataset and in this, 79% of applications were classified as being good risk - 10% higher than everything in the dataset.
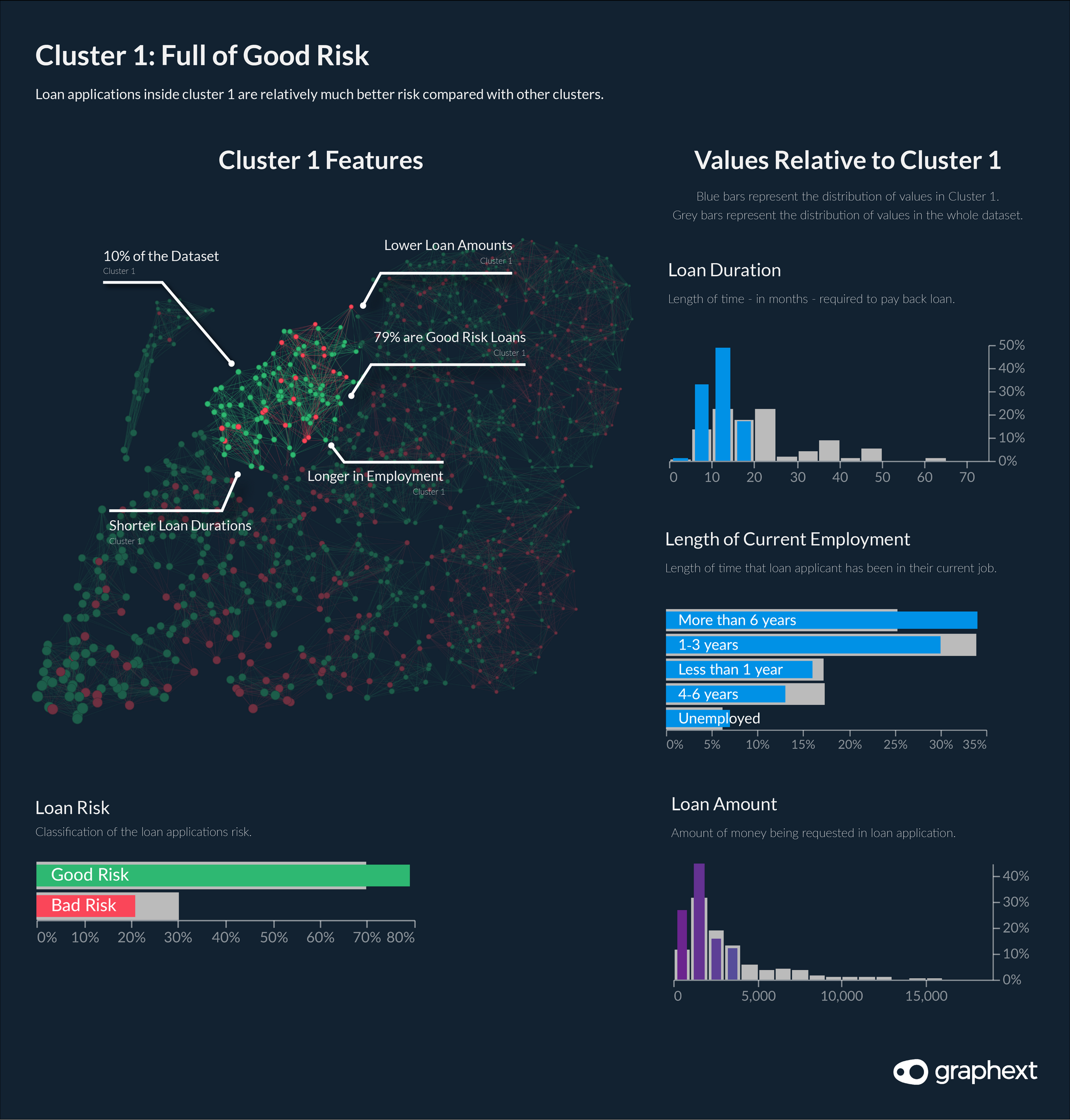
Cluster 1: The features of a good risk cluster
This cluster was predominantly made up of people in their thirties who were single. I found the fact that being single was related good risk very interesting. I would consider that two incomes would be better than one - however this finding may be influenced by a lower likelihood of a married couple having two incomes in the 1970s.
The features of cluster 1 confirmed a few suspicions I was beginning to have about the dataset. Loan applications here were for short durations and low amounts. Additionally, the purpose of the loan was to buy either a TV or radio. Can you imagine taking a loan to buy a radio these days?
Social vs Financial Factors
The characteristics of a loan application can be seen as either financial or social factors. Age and status are variables relating to an applicant's social status, whereas savings account and loan duration are variables relating to their financial status.
Social Factors
Selecting bad risk loan applications and inspecting their features suggested that women were more likely to receive bad risk ratings. The same is true for younger people, especially loan applicants under the age of 26.
It was also interesting to note that homeownership helped to increase the positivity of an applicant's risk profile. Tenants or those in free housing were more likely to receive a bad risk rating.
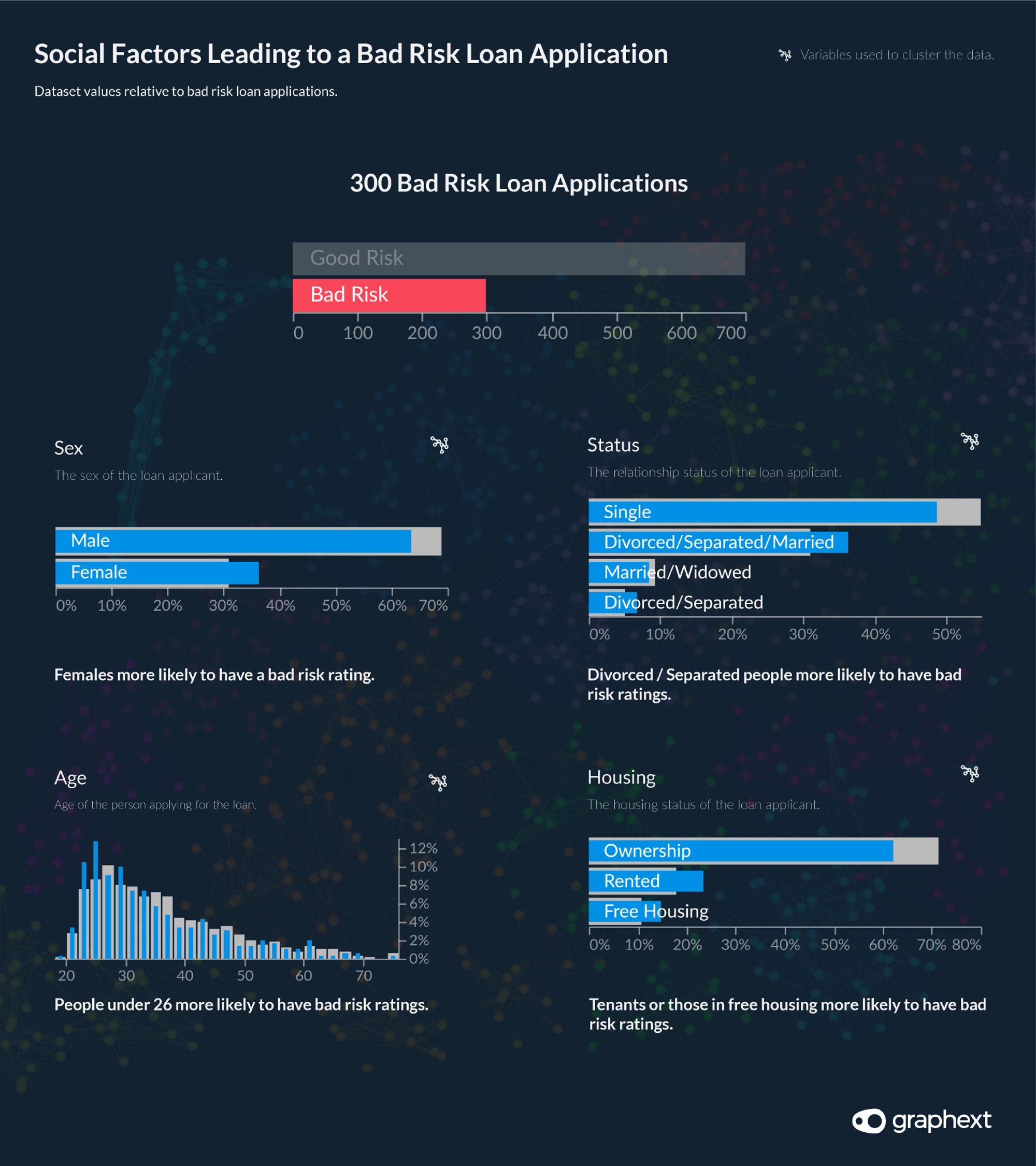
Variable Charts: The social factors related to bad risk loan applications
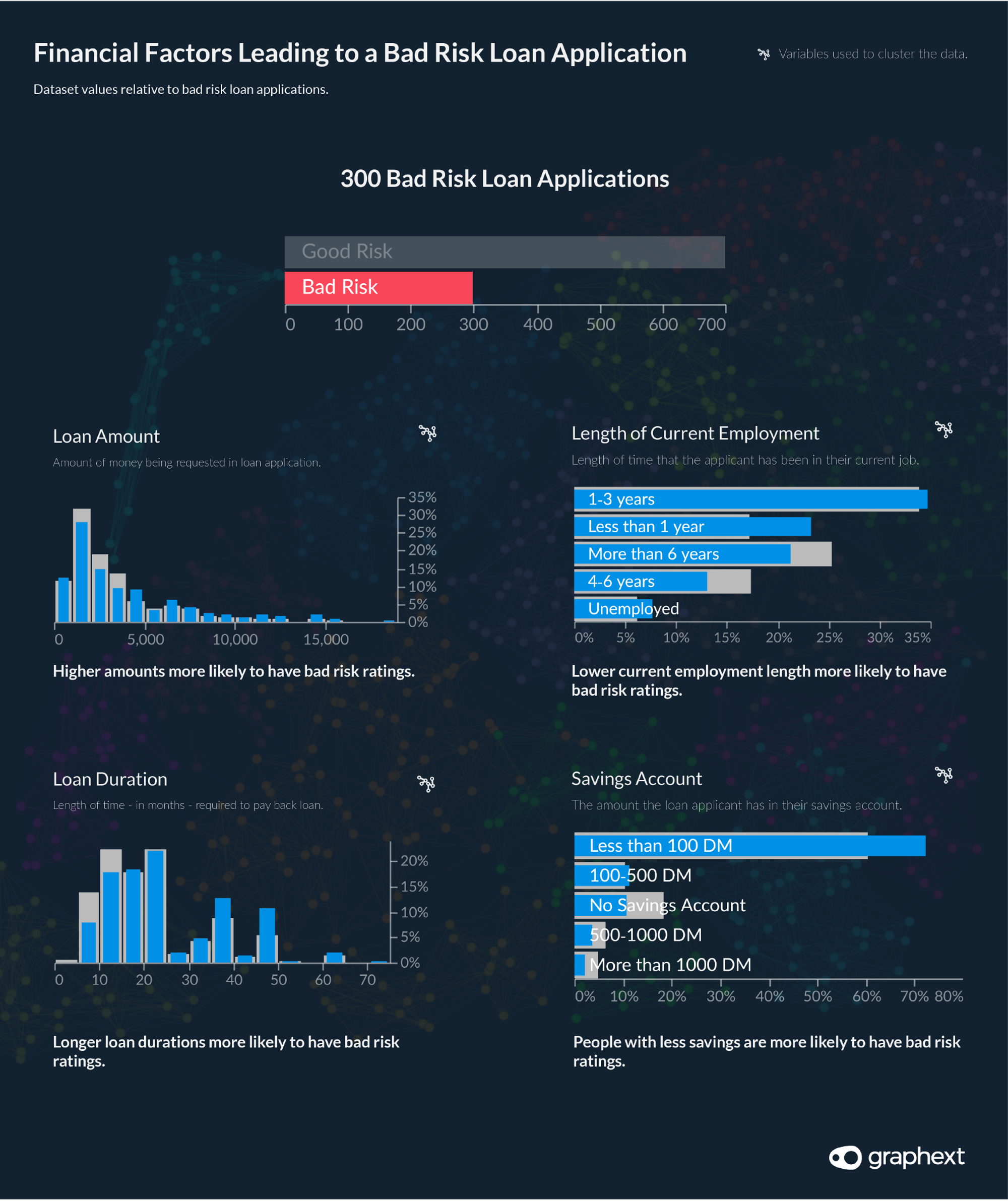
With women and younger people coming out worse, I sincerely hope brokers and banks have changed their attitude changed since 1970. It would make for an interesting comparative study to find out just how much of this bias still applies.
Financial Factors
I am less persuaded that the influence of financial factors will have changed over the past half a century. Longer loans for higher sums of money are profiled as bad risk - and this makes sense! Not only this, but applicants with more savings and longer periods of current employment are more likely to be awarded good risk profiles.
Variable Charts: The financial factors related to bad risk loan applications
The ideal candidate to receive a bank loan in 1970s Germany would be a single man who already owns their own property and is over 32 years old.
So, with all this in mind, the ideal candidate to receive a bank loan in 1970s Germany would be a single man who already owns their own property and who is over 32 years old. He would have been in his current job for a sustained period and would have a reasonable amount of savings. Furthermore, he would be looking for a low sum of money over a short payback period.