2021-10-26
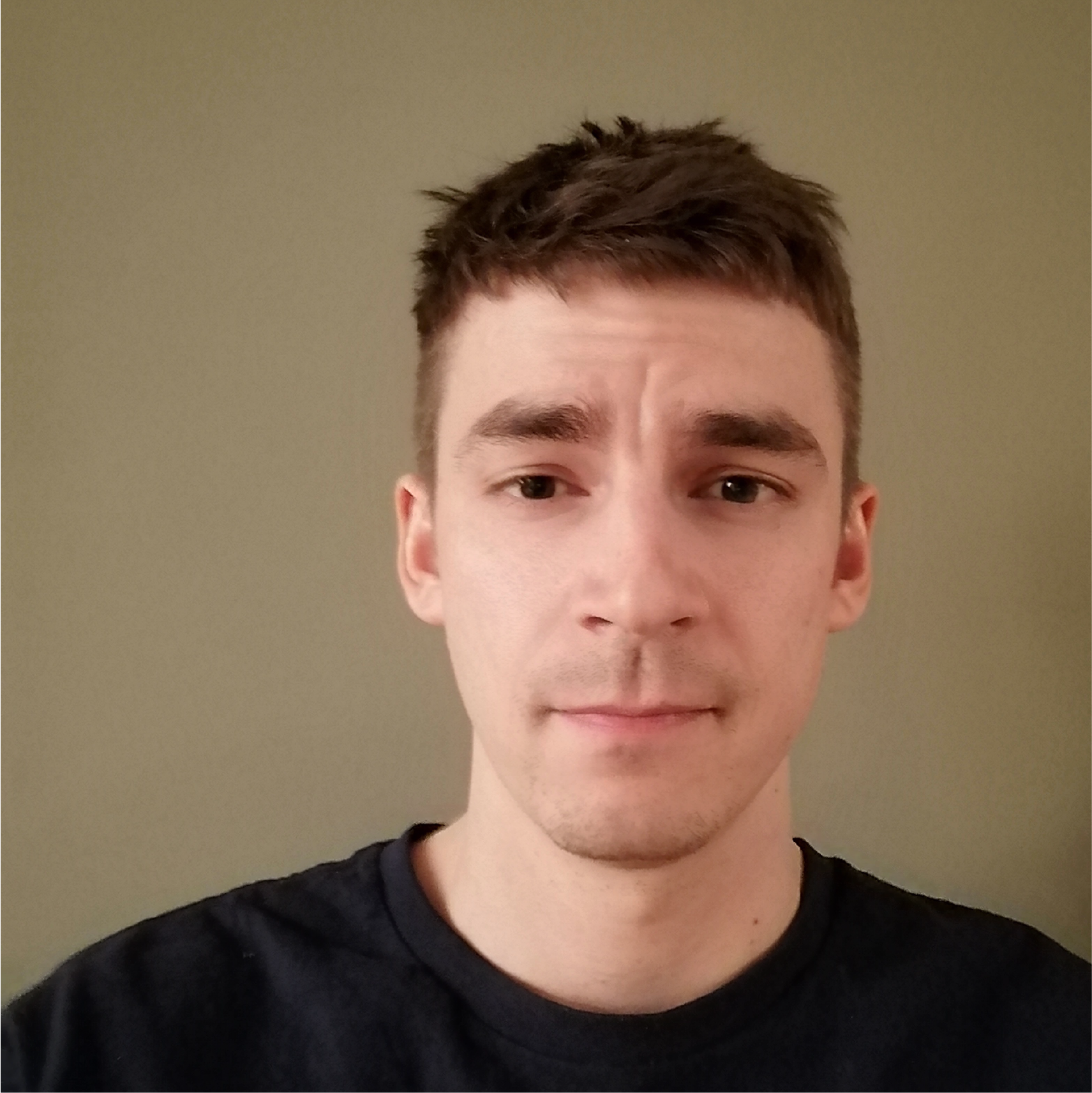
Andy Clarke
How to Perform Simple & Effective Customer Segmentation | A Walkthrough with Data from a Delicatessen (Dataset interactive)
Customer segmentation involves splitting a customer base into distinct groups. These customer segments are defined by specific and shared characteristics, behaviours or preferences that help businesses to spot patterns and associate customers with one another. This article walks through the steps involved in a simple customer segmentation analysis. Using sales data from a delicatessen, we'll segment customers according to their buying preferences and behaviour. To achieve this, we'll use a powerful machine learning technique known as clustering.